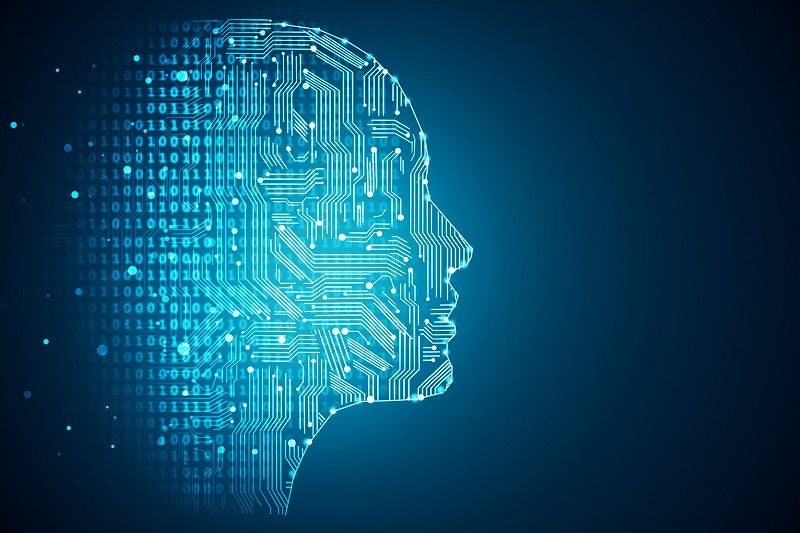
There’s little doubt that the human brain is an incredibly powerful tool. However, it is not without its limitations. Cognitive biases and their attempts to simplify the stacks of data and information we are exposed to every day can impair our ability to make fair judgements.
According to Pete Davies, head of digital engineering solutions at Uniper: “When humans begin to know somebody or something too well, biases begin to form in our minds. As the information we receive grows, so too does the bias, having an inevitable influence over the decisions we make.
“As an example, ‘Tim’ is a quiet individual who is well read, considered in his responses and likes to spend time with small groups of friends or on his own. Is Tim more likely to be a librarian or a truck driver?
“Most people will say librarian because they’ve been given extra information. But the reality is, Tim is more likely to be a truck driver, simply because there are statistically more truck drivers in the world.”
The benefits of machine learning
Examining the way in which machine learning (ML) can combat the effects of human bias in court case bail decisions, a 2017 study used a large set of data from cases spanning 2008 to 2013, with scientists feeding the same information available to judges at the bail hearing into a computer-based algorithm.
The dataset included the offender’s current offence, their prior criminal records, their age, and any re-arrests prior to historic case resolutions, and excluded factors such as race, ethnicity or gender. Defendant age, however, was the only demographic information given.
The study found that the use of algorithms could reduce jail population by 42% and crime by up to 25%, simply because the computer did not experience bias when deciding which defendant was a flight risk. While the machine only had access to objective data during the decision-making process, the judges met with defendants face to face. This meeting gave them an initial impression of the person, thus adding to their information set and allowing human bias to kick in.
Davies continues: “For the majority of people, when we interact with others, we look to believe in what they say. This is for evolutionary purposes and aids in building the support, trust and friendships we need to feel a sense of safety in numbers or belonging.
“We cannot remove this bias, so when given too much or perhaps sporadic information, we tend to apply too much weight to its importance and then make poor decisions. This is the opposite of computers. When you provide information to computers, they will treat it consistently or at least relatively, compared to humans.”
When a computer is not given sufficient information, however, the machine is unable to make reasonable conclusions. In the bail case study, which included more than 550,000 records, an in-depth understanding of the datasets could be obtained.
Advanced condition monitoring
How data is best utilised by machine learning, as well as the effects of human bias, is integral to understanding how man and machine can successfully work together. At global energy supply company Uniper, for example, advanced condition monitoring (ACM) has been carried out over the last decade. In that period, the company has recorded every fault detected and validated at a power plant. While not actual failures because they were caught early enough, these pseudo failure mechanisms can still provide essential, actionable insights.
“We take this history and feed it an ensemble of ML/AI algorithms to automatically identify faults and provide a diagnosis,” says Davies. “Whilst it isn’t bulletproof, it’s a good example of machines and humans working together to create large sets of data and removing biases from the decision making process, without completely taking humans out of the process.
“The final human intervention of validating actionable alerts before being issued to a site is important. When running a power plant, safety is rule number one and having a machine run wild by making decisions about operations or maintenance independently is dangerous.
“We know the industry is changing. Assets are closing; people are moving into different sectors or starting new careers, and so to combat our declining knowledge base in the power industry we must turn to machine learning and AI to help us wherever we can.”