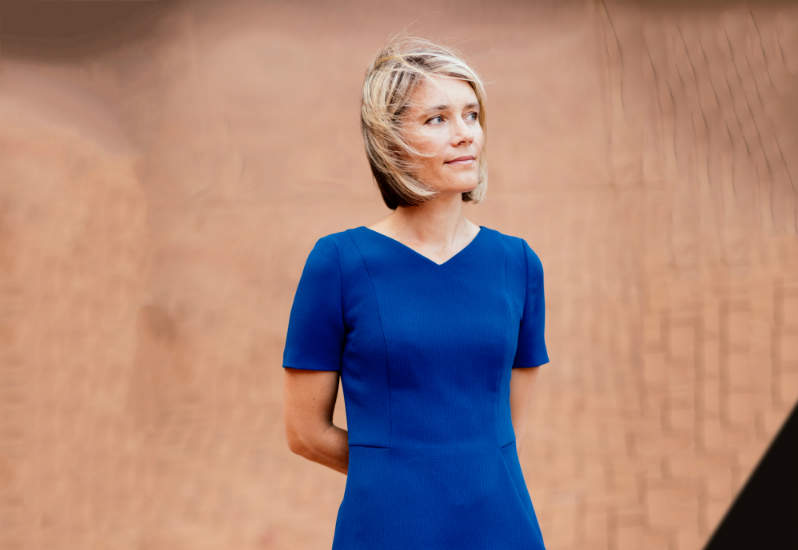
US energy policy has been in flux over the last few years, from the infamous withdrawal from the 2015 Paris Agreement on climate change last year, to President Trump’s commitment to the American coal industry and California Governor Jerry Brown’s mid-September signing of executive order B-55-18 pledging California to carbon neutrality by 2045. The state of US energy, and indeed that of the rest of the world, is increasingly difficult to predict.
Thankfully, the work of experts such as MIT associate professor of energy studies Jessika Trancik and her team makes the future much easier to read. Trancik evaluates different energy technologies and systems for the reduction of carbon emissions and other greenhouse gas emissions from energy and energy-use sectors, creating quantitative data models to understand the future energy market, and to inform technology design and policy decisions. Here Trancik explains the importance of effective data models and their broader impact.
How well do you really know your competitors?
Access the most comprehensive Company Profiles on the market, powered by GlobalData. Save hours of research. Gain competitive edge.
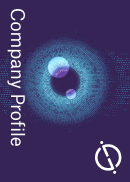
Thank you!
Your download email will arrive shortly
Not ready to buy yet? Download a free sample
We are confident about the unique quality of our Company Profiles. However, we want you to make the most beneficial decision for your business, so we offer a free sample that you can download by submitting the below form
By GlobalDataElliot Gardner: Since US energy policy has renewed its focus on coal, is it more important to focus on making traditional carbon generation technologies more sustainable or should we focus on green renewable alternatives?
Jessika Trancik: I think it’s important to do all of the above. Of course there’s always limited time and financial resources, so you want to choose your efforts carefully, and that’s where measuring the impact of different efforts comes in.
The goal of my research is to help us do this, to make these decisions more systematically, based on data and a quantitative comparison of the impact of different technologies. We may say that natural gas is relatively low carbon, but how low carbon can it be and how does it compare to other alternatives? What percentage of natural gas would we want to use as part of the energy mix? And for how long? Trying to pin down the magnitude of the impacts of different technologies, and the scale of the contribution that they can make to address climate change is something I think is really important.
EG: How do you compare the data from many different forms of energy generation – coal, nuclear, renewables – in a usable way for the energy industry at large?
JT: One of the tricky problems is the question of how you compare methane emissions to carbon dioxide emissions. What’s typically done is to use a factor that converts grams of methane to grams of CO2 equivalent emissions. But when you look at how that’s actually done and get into the details, you can see that the answer you’re getting is going to be very sensitive to that conversion factor that you’re using.
Most commonly, almost universally, the conversion factor that’s used is based on a global warming potential over 100 years, so it’s the contribution of one unit of methane against one unit of carbon dioxide. But that choice of 100 years is actually an arbitrary one. When it was first proposed, this metric was never intended to be one that would be used in policy and so forth. If you vary that time horizon from 100 years, the values can change very significantly.
If you vary it to 50 years, or 25 years, all the way making an instantaneous comparison, you can see that that advantage of natural gas over coal can be cut in half. So then the question comes up of what metric should we use? What we propose is that the time horizon can be based on a climate change mitigation goal, so if you have a given year by which you’re trying to stabilise radiative forcing overall, then you can base your time horizon on that stabilisation year, and that time horizon can change as you approach that year, meaning that the impact of methane against CO2 would actually increase. One unit of methane admitted today is not going to have the same impact relative to CO2 as one unit admitted in ten to 20 years from now.
So yes, that conversion factor would be changing over time, but what it allows you to do is to avoid unintended overshoots of emissions policy targets.
EG: Will recent governmental policy decisions and commitments have a significant impact on the energy market?
JT: It’s not really the government that makes those decisions in any case. Policies are obviously important, but it’s the private sector, consumers and various non-governmental actors that really play an important role in what decisions get made. For example, many have said this before me, but it’s not really possible for the US Government to make a decision on whether or not the coal industry will see resurgence.
Of course, those policy decisions still do have a real impact on what happens in the industry. If, for example, you’re not regulating the release of methane emissions from natural gas plants and you’re allowing the least efficient coal plants to continue operating, then of course that’s going to reduce the operating cost for those more polluting power plants – but at the end of the day there’s still the recognition in the marketplace from private companies and consumers that things are moving in the direction of regulating emissions overall around the world.
It’s not necessarily happening very quickly, but that’s the direction things are moving in, and that’s something that players within the market take into account, so even if regulations are relaxed – and you see this with vehicle emissions standards – there comes a point that regulations are relaxed so much that there’s uncertainty about what will happen in the future, and that just brings about a lot of confusion, and industry tends not to like that either.
EG: Is it tricky to factor future technology into energy data models?
JT: Definitely, but for some technologies there is data on how they’ve evolved in the past. That helps you develop statistical models, much like you would do in finance. You can develop a model to understand the uncertainty related to that forecast.
Now, in some cases – such as with carbon capture and storage – we have very little data on cost, so that would be an example of a technology where the full lifecycle costs are very uncertain, which is again something you would want to take into account in making decisions. That adds to the risk and uncertainty of that area of your portfolio.
Another example is photovoltaic cells. If you look back at some models and forecasts over the last ten or even 20 years, you can see in some cases the rates of change in the cost of photovoltaics were not predicted, and that meant that the forecasts were really far off. Over 40 years we’ve seen a two order of magnitude drop in cost, which is really large. You can imagine back 40 years ago if your simulation model wasn’t taking that into account, then your answer for a least-cost energy system would be very far off. It really does demonstrate the importance of taking into account technological change, and that future change can be very uncertain.